Business
29 Points to Consider for Private Enterprise LLMs
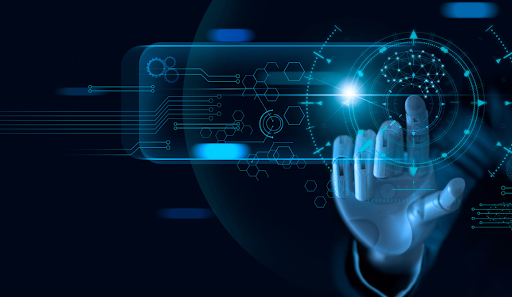
Navigating the cutting-edge intersection of technology and legal services, Private Enterprise Legal Language Models (LLMs) are reshaping the approach businesses take towards legal document management and interpretation. With 29 critical points to consider according to Iterate.ai, this framework aims to guide businesses through the intricate process of integrating LLMs into their operations. These considerations span from security concerns to technological capabilities and scalability, ensuring that businesses can leverage LLMs effectively while navigating the complex legal terrain.
According to Iterate, successful private LLMs will have certain qualities, such as model superiority, a nice and addressable market, a sustainable source of data and a path to independence. Underlying AI needs to be better, faster, or cheaper than existing general-purpose models for the service provided. LLms need to solve a widespread yet specific problem while using a sustainable source of data, which is key for continuously improving the underlying model and staying ahead of the competition. Finally, being under the thumb of Model as a Service is not ideal in the long run. If AI is the core of your business, having full control over the data/model should be a major goal long-term.
The 29 Points to Consider
- An LLM trained specifically on the enterprise’s own use cases is better.
- Superfluous information lessens accuracy.
- LLMs can become Intellectual Property.
- Source data must be secure.
- Data needs guardrails.
- External training data sets are discouraged.
- Model Security is equally important.
- Adopt a platform, not just a model.
- Beware of corporate machinations.
- Hosting/IT/Network Security applies to LLMs.
- LLMs can be measured.
- Latency is crucial.
- Model switching in a private LLM may improve performance vs retraining on a public LLM.
- Both public and private LLMs benefit from increased context window size.
- Hallucination control is easier in a private LLM (vs methods for a public model).
- Public LLMs are limited in hallucination control.
- Token Cost is a factor.
- LLMs that can run on a CPU are vastly less expensive.
- Vendor lock-in is prevalent with model-as-a-service.
- Vendor stability might prefer the mid-market.
- Open Source models will be more flexible.
- Model output flexibility should be considered.
- Fine-tuning should be monitored just like any other application.
- AGI is coming, but that may not matter as much as the press.
- Data Source sustainability is essential.
- Synthetic data helps train models but is not a sustainable solution.
- Legality risks will continue.
- Internal human training is key.
- Support capacity for a private LLM involves several factors.
The emphasis on training LLMs specifically for an enterprise’s unique legal challenges highlights an economic transition to more customized solutions. This customization allows enterprises to focus on the nuances of their legal environment, minimizing the noise of superfluous information that could detract from the model’s effectiveness. The potential for LLMs to evolve into proprietary Intellectual Property not only adds strategic value to the enterprise but also secures a competitive edge in the marketplace. The emphasis on secure source data and the necessity for stringent data guardrails further accentuates the critical balance between leveraging advanced AI capabilities and ensuring ethical and secure data practices.
These points address the operational and strategic considerations of deploying LLMs within private enterprises and highlight the multifaceted nature of this technological adoption. The caution against reliance on external training datasets and the importance of model security reflect the complex landscape of legal compliance and data privacy. The recommendation to adopt a comprehensive platform rather than a singular model suggests a holistic approach to integrating LLMs, encompassing not just the technology but also the supporting infrastructure and practices. This includes considerations around hosting, IT security, and the potential pitfalls of corporate machinations. The discussion on the cost-benefit analysis of model deployment, particularly the advantages of CPU-run LLMs over more expensive alternatives, and the warning against vendor lock-in, offer practical insights for businesses aiming to navigate the economic aspects of LLM integration. These considerations collectively provide a roadmap for enterprises seeking to harness the power of LLMs in a manner that is secure, efficient, and strategically aligned with long-term business goals.