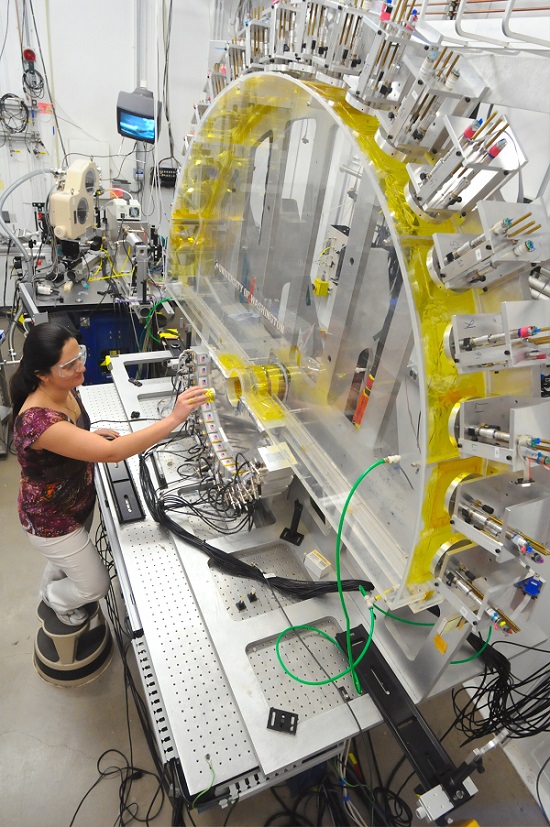
By Dawn Fitzgerald, the AI Trends Insider on Executive Leadership
[Editor’s Note: Today we are introducing a four-part series on AI Holistic Adoption for Manufacturing and Operations, written by Dawn Fitzgerald from an executive leadership perspective. The columns are to include key execution topics required for the enterprise digital transformation journey. Planned topics include: Value, Program, Data and Ethics.]

The Executive Leadership Perspective
Successful Digital Transformation and AI adoption requires executive leaders to ensure that AI solutions are bringing Value to the enterprise. Here, Value means that these solutions must be monetizable, sustainable and scalable. Monetizable means able to be turned into cash in the business world; in this context it means to become a source of profit.
For the AI solutions to be monetizable, we must provide measurable value in the near term. Monetization can be in the form of workflow improvements and efficiencies which translate into cost savings, or customer feature enhancements which translate into sales.
Once AI solutions are launched, we protect our investment by ensuring that they are sustainable for industrialized applications, and scalable for future growth opportunities either within our organization or within the product line. Broader strategic industry positioning goals are necessary motivators but not sufficient for successful enterprise AI adoption.
Real Value achieved with Value Analytics approach
The allure of AI and Machine Learning is strong because of its promise of increased capabilities, efficiency and speed. We must however be aware of the temptation to launch AI solutions based on trends without real value add, maybe based on hype. As executives, we are especially susceptible to this risk if our organizations have defined an enterprise strategy to “take an AI leadership position” in our industry. Under these scenarios, data science teams are frequently asked, “What can you do?”, “What do you have for this industry segment?”.
While these are important questions and the input from these skilled teams is highly valuable, it is truly a bottoms-up approach. It must be coupled with a top-down perspective from your strategic marketing teams and industry segment Subject Matter Experts. These experts must bring the focus on where the customer pain points reside and frame these requirements, identifying gaps that could potentially be addressed by AI solutions. The cross functional team then identifies high value targets for potential AI solutions.
It is critical to the success of enterprise AI adoption that the executive leadership take a Value Analytics approach to AI adoption. This means that AI solutions must include identifying user pain points, user stories, a broad analytics algorithmic inclusion, quality criteria and identify success criteria with key analytic value metrics.
A Broad Analytics approach brings Higher Value
To derive higher value and adoption from your AI solutions, it is important to embrace a broader analytics view. This view includes a broader set of analytics (AI/ML algorithms, statistics, observation and reporting) and combinations of ML algorithms to provide measurable value to the end user/customer.
The unique problems of manufacturing and operations environments frequently require the combination of analytics components to optimize the AI solution. These issues include high site to site variation, high human interaction influence, hostile environment and small data problem considerations. As highlighted in the MIT Machine Intelligence for Manufacturing and Operations presentation by MIT’s Prof Duane Boning, research accentuates the need to apply the correct Machine Learning methods or combination of methods to targeted Manufacturing and Operations Use Cases.
Metrics of Value Analytics
Results Measurement is a critical tool for executive leaders to manage their businesses. Focused Results Measurement is mandatory for executive leaders that are driving digital transformation and AI solutions in their organizations. The Value Analytics approach demands a definition of the pain point to be resolved along with user stories and a clear measurement of success.
Clear Success Criteria must be defined before the AI solution efforts begin. This ensures monetization results vs exploratory research. Executive leaders need to frame these requirements for the marketing and development teams executing the AI solutions.
Plant and Grow the Seed
To achieve the goals of enterprise Digital Transformation and AI adoption, it is critical to start small. The key to success is to identify pointed, isolated, and high value targets for the AI solution. For example, predictability of machine ‘X’ failures or optimization of key manufacturing line parameter ‘Y’. Then, while designing the associated Analytics Program for these targets, the design must also keep the long term, larger scale goals of the Program in mind. The executive leadership must guide their team to design and launch a small Analytics Program with the capability to scale. Goals of larger scale adoption that come into play include expanding the scope of high value targets and expanding the number of teams in the enterprise that address high value targets with AI. Thinking big requires an Analytics Program design that spans all components of AI Holistic Adoption.
Components of AI Holistic Adoption
Defining the Value of AI solutions and realizing the Value of these solutions are two very different things. A recent IDC report on a survey of global organizations that are already using AI solutions found only 25% have developed an enterprise-wide AI strategy. Most organizations want to adopt AI; however, it is much more complex than simply applying a ML algorithm to a data set.
Successful AI adoption is the combination of people, process and tools. This is Holistic Adoption. The people included are not only the designers, but the entire stakeholder base associated with the AI/ML solution in question. Business processes will need adjustment and tools for stakeholders will need to be part of the evolution of the digital culture. All of these components of AI Holistic Adoption must be addressed in the Analytics Program.
Dawn Fitzgerald is Head of Innovation, Platforms and Analytics for Schneider Electric, where she is focused on the development of new digital services. She is experienced with how to make a range of AI technologies work for multi-site, global organizations. Learn more at LinkedIn.