Artificial Intelligence
AI Tools Will Help Us Make the Most of Spatial Biology
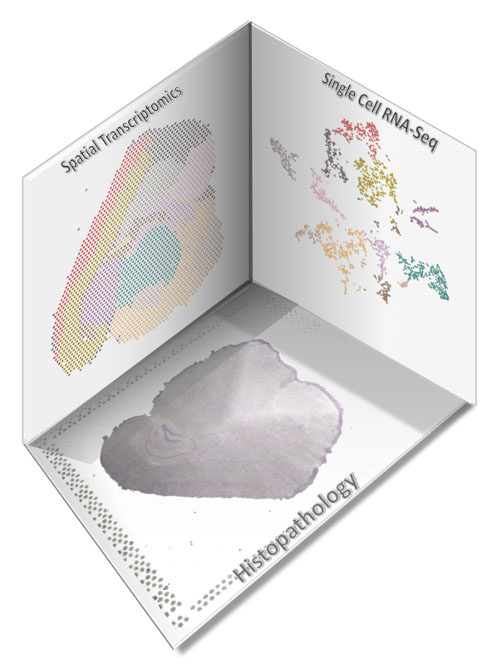
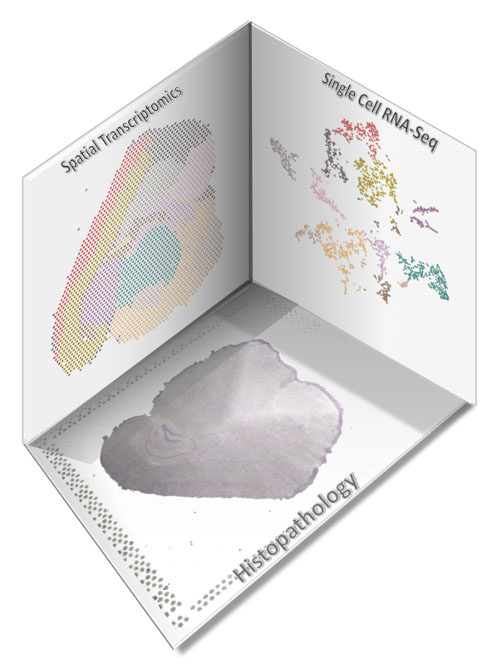
Contributed Commentary by David W. Craig, Ph.D. and Brooke Hjelm, Ph.D.
We have heard a lot about cellular and tissue spatial biology lately, and for good reason. Tissues are heterogeneous mixtures of cells; this is particularly important in disease. Cells are also the foundational unit of life, and they are shaped by those cells proximal to them. Not surprisingly, the research field sought to survey cellular and tissue heterogeneity. The last decade saw massive adoption of single-cell sequencing RNA. This approach requires that we disaggregate cells, leading to accounting and characterization of cell populations, but at the same time losing their spatial context such as their proximity to other cells or where they fit with traditional approaches such as histopathology.
Enter Spatial Genomics
That’s why we have welcomed spatial transcriptomics and a focus on mapping RNA transcripts to their location within a tissue. After all, understanding disease pathology requires that we understand not only the underlying genomics and transcriptomics but also the relationship between cells and their relative locations within a tissue. Along for the ride: new avenues for the study of cancer, immunology, and neurology, among many others. What’s changed is the emergence of new tools for resolving spatial heterogeneity. SeqFISH and MerFISH are novel approaches for mapping gene expression within model systems. Multiple companies such as 10x Genomics and NanoString are now democratizing access to spatial transcriptomics, introducing new technologies and assays. They are opening up the study of disease pathology.
AI & Deep Learning: Adding to Our Vocabulary
New experimental methods often start with historical analysis approaches. Let’s consider the first step in analysis: finding clusters of spots/cells with similar gene expression and then visualizing by reducing dimensions. In single-cell RNA-seq, the tSNE projection and color-coding clustering may be the signature plot, much like the Manhattan plot was to the GWAS.
Yet, critically, we haven’t leveraged the underlying histopathology image—the foundation of diagnosis and study of disease. We haven’t leveraged the fact that two spots are neighboring. What happens when we do? What happens at the edges between two clusters? What happens when cell types intersperse or infiltrate, such as in immune response? Are there image analysis methods we aren’t considering that have a high potential impact?
Indeed, concepts such as convolutional neural networks (CNNs) and generative adversarial networks (GANs) have been instrumental in classifying features and underlying hidden layers. We can go beyond the tSNE in spatial transcriptomics—and the question should be about viewing the latent space (the representation of the data that drives classifying regions and the discovery of hidden biology). These terms and concepts are foundational when it comes to artificial intelligence and need to be front and center in spatial transcriptomics analysis.
Of course, the use of AI and deep learning terminology is ubiquitous. Getting away from the hype, from self-driving cars to the successes in image recognition (ImageNet Challenge), some of the most remarkable achievements leverage spatial and imaging data. Data matters and one then asks: should we consider a single spatial transcriptomics section as one experimental data point, or is it 4,000 images and 4,000 transcriptomes?
In spatial biology, we can anticipate that applying AI to cell-by-cell maps of gene or protein activity will pave the way for significant discoveries that we might never achieve on our own. Incorporating spatially-resolved data could be the next leap forward in our understanding of biology. There will be questions we never even knew to ask that may be answered by combining spatial transcriptomics and spatial proteomics. But to get there, we need to come together and work as a community to build up the training data sets and other resources that will be essential for giving AI the best chance at success.
We have yet to truly make the most of the spatial biology data that has been generated. If we do not address this limitation, we will continue to miss out even as we produce more and more of this information.
David W. Craig, PhD ([email protected]), and Brooke Hjelm, Ph.D. ([email protected]) are faculty within the Department of Translational Genomics, University of Southern California Keck School of Medicine.