Technology
Data Science in Financial Risk Management–What You Need to Know
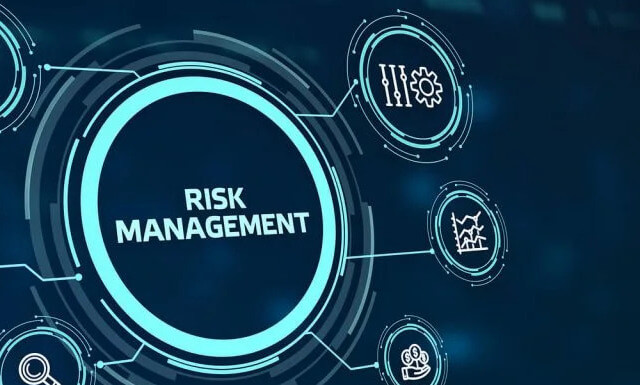
Introduction
Data science plays a crucial role in financial risk management, helping institutions identify, assess, and mitigate various types of risks. In commercialised cities where technological awareness is high, data science techniques are increasingly being used as the means for countering the perennial problem of risk management that besets the financial sector. Thus, a Data Scientist Course in Pune Or Mumbai that is tailored for the financial domain will provide extensive coverage on using data-driven techniques for combating fraud.
The Role of Data-Driven Techniques in Risk Financial Management
Here are some important ways in which data science can help with financial risk management:
- Types of Financial Risks: Financial institutions face several types of risks, including credit risk, market risk, liquidity risk, operational risk, and systemic risk. Data science techniques can be applied to analyse data related to each of these risk types, allowing institutions to quantify and manage their exposure effectively.
- Data Collection and Management: Data science relies on the availability of high-quality data for analysis. Financial institutions collect vast amounts of data from various sources, including transaction records, market data, customer information, and economic indicators. Data scientists play a crucial role in collecting, cleaning, and organising this data to ensure its accuracy and reliability for risk analysis. These are the essential first steps in data analysis. However, these steps have a direct say on the effectiveness of the final recommendations and findings of an analytical initiative and therefore form an important topic in any Data Science Course .
- Predictive Modelling: Predictive modelling is a key component of financial risk management, allowing institutions to forecast future outcomes and identify potential risks. Data science techniques such as machine learning and statistical modelling can be used to develop predictive models for credit risk assessment, market volatility prediction, and customer behaviour analysis.
- Scenario Analysis and Stress Testing: Data science enables financial institutions to conduct scenario analysis and stress testing to assess their resilience to adverse events and market conditions. By simulating various scenarios and stress-testing their portfolios, institutions can identify vulnerabilities, evaluate capital adequacy, and develop contingency plans to mitigate potential losses.
- Fraud Detection and Prevention: Data science techniques such as anomaly detection, pattern recognition, and network analysis are used to detect and prevent fraud in financial transactions. By analysing transaction data and identifying unusual patterns or suspicious activities, institutions can minimise the risk of fraudulent behaviour and protect their assets and customers from financial crime. Most financial institutions, instead of hiring dedicated technology experts, choose to upskill their financial professionals by conducting in-house courses in data sciences for them or sponsoring their enrolment for data science bootcamp training sessions or a classroom Data Scientist Course.
- Regulatory Compliance: Financial institutions are subject to regulatory requirements and reporting standards aimed at ensuring financial stability and consumer protection. Data science plays a crucial role in regulatory compliance by helping institutions analyse and report relevant data, monitor compliance with regulatory requirements, and assess the impact of regulatory changes on their operations. With regulatory compliance laws becoming increasingly stringent and severe legal encumbrances being imposed for non-compliance, the legal aspects of handling data form mandatory part of the curriculum on any Data Scientist Course.
- Model Validation and Governance: It is essential for financial institutions to validate and govern their risk models to ensure their accuracy, reliability, and compliance with regulatory standards. Data science techniques are used to validate models, assess their performance, and identify potential biases or weaknesses. Robust model governance frameworks help ensure that risk models are used appropriately and effectively in decision-making processes.
Conclusion
Overall, data science has revolutionised financial risk management by providing institutions with advanced analytical tools and techniques to assess and mitigate risks more effectively. As financial markets continue to evolve and become increasingly complex, the role of data science in risk management will become even more critical in safeguarding the stability and integrity of the financial system. The financial sector in tech-savvy cities prefer their professionals to be equipped with skills in data science technologies, which is why a Data Scientist Course in Pune or Mumbai find as many enrolments from financial professionals as from professionals who are more directly involved in technological roles.
Business Name: ExcelR – Data Science, Data Analyst Course Training
Address: 1st Floor, East Court Phoenix Market City, F-02, Clover Park, Viman Nagar, Pune, Maharashtra 411014
Phone Number: 096997 53213
Email Id: [email protected]