Artificial Intelligence
Decoding Explainable AI: Revolutionizing Financial Institutions
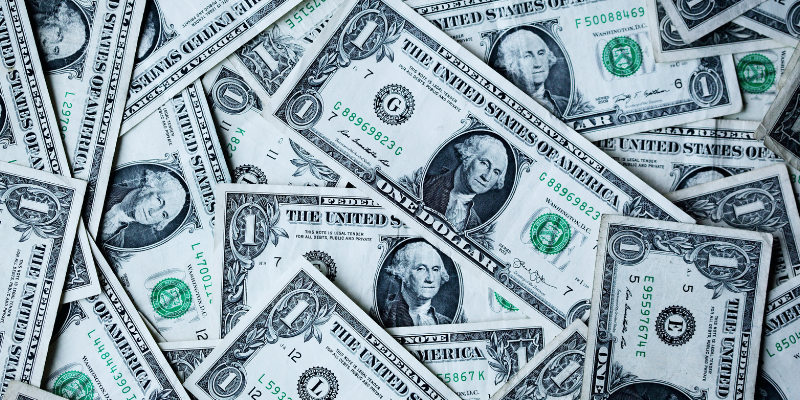
Artificial intelligence (AI) has revolutionized financial institutions, enhancing decision-making processes with its speed and accuracy. However, the lack of transparency in AI algorithms has hindered widespread adoption, leaving professionals puzzled about how decisions are made. Fortunately, explainable AI has emerged as a game-changer, unraveling this mystery and opening up new possibilities for financial organizations. In this blog post, we will explore the concept of explainable AI, its significance in the finance industry, the benefits it offers, challenges in adoption, real-world examples, and tips for implementation.
Introduction:
The rise of artificial intelligence (AI) has transformed how financial institutions operate, bringing about faster and more accurate decision-making processes. However, a significant challenge has been the lack of transparency in AI algorithms, leaving professionals puzzled about the reasoning behind AI decisions. But fear not! The advent of Explainable AI (XAI) has emerged as a groundbreaking solution, promising to unravel this mystery and revolutionize the finance industry. In this article, we will explore what Explainable AI is, why it’s important for financial institutions, its benefits, implementation challenges, real-world examples, and practical tips for successful adoption.
What is Explainable AI?
Explainable AI refers to the ability to provide justifications for the results produced by an AI system. It involves understanding the decision-making process of the machine. In fields like finance, healthcare, and law enforcement, where critical decisions are based on AI predictions, explainable AI is crucial. Two main approaches to explainable AI exist: model-based and model-agnostic. Model-based methods create an interpretable model that mimics the behavior of the original AI system. Model-agnostic approaches analyze the output of the complex AI system to understand its workings. Each approach has advantages and disadvantages, and financial institutions must consider these factors when choosing the right approach.
Importance of Explainable AI in the Finance Industry
Explainable AI holds immense importance in the finance industry for several reasons.
- Financial institutions rely increasingly on AI for decisions regarding lending, investing, and other financial products. Understanding how and why AI makes these decisions is critical to avoid costly mistakes.
- Explainable AI aids compliance with regulations like the Gramm-Leach-Bliley Act and the Dodd-Frank Wall Street Reform and Consumer Protection Act. These regulations require transparency in disclosing the factors influencing creditworthiness and pricing decisions. Explainable AI facilitates transparent and understandable communication of this information to consumers.
- Explainable AI builds trust between financial institutions and customers. In an era of data breaches and identity theft, customers are concerned about sharing personal information. However, if they understand how AI uses their data, they are more likely to trust the institution.
- Improves customer service by providing guidance to customers who are denied loans or given higher interest rates. Moreover, customer service representatives can use explainable AI to quickly address customer inquiries, enhancing overall service quality.
Benefits of Using Explainable AI
Using explainable AI offers numerous benefits for financial institutions. It enhances customer experience by providing insights into behavior and preferences, enabling institutions to design better products and services. Additionally, it helps in fraud detection and prevention by identifying suspicious patterns in financial transactions. Compliance with regulations is also facilitated through transparency in AI decision-making processes. Explainable AI aids institutions in better understanding their data and the relationships between different data points.
Challenges in Adopting Explainable AI in the Financial Sector
Several challenges accompany the adoption of explainable AI in the financial sector. Financial institutions tend to be risk-averse, often reluctant to adopt unfamiliar technologies. Moreover, explainable AI systems can be complex and expensive to develop and deploy. Ensuring regulatory compliance and meeting all requirements can be challenging as well.
Examples of Explainable AI in Practice
Various examples demonstrate the application of explainable AI. One such example involves a system predicting loan repayment. By analyzing borrower data like credit scores and employment history, the system produces a score indicating the likelihood of repayment. This system is explainable since lenders can understand the basis of the prediction and make informed decisions regarding lending.
Another example is a system used to identify fraudulent activity by examining financial transaction data. Based on factors like transaction amount and location, the system generates a fraud likelihood score. Financial institutions can understand why the system made its prediction and take necessary actions to prevent fraud.
Tips to Implement Explainable
Implementing explainable AI in the finance industry can be transformative. Here are some tips for successful implementation:
Be transparent: Communicate the role of AI in your business to customers and stakeholders, fostering trust and understanding.
Explain the system: Provide insights into the algorithms and data sources used, enabling comprehension of AI decisions.
Use human-readable explanations: Avoid technical jargon and employ natural language explanations to enhance understanding and build trust.
Offer user control: Allow users to select the level of explanation they desire, catering to individual preferences.
Continuously improve: Utilize user feedback to enhance explanation quality over time, recognizing that explainable AI is an evolving field.
Conclusion
Explainable AI is crucial for financial institutions to ensure transparent and effective decision-making. By leveraging explainable models, financial institutions can make better use of data, enhance operational efficiency, reduce risk exposure, and provide more reliable services to customers. Embracing explainable AI empowers financial institutions to monitor compliance, protect against risks, and make data-driven decisions.