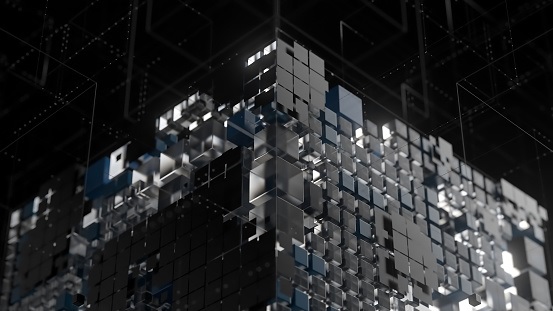
By AI Trends Staff
With the energy and compute demands of AI machine learning models trending at what appears to be an unsustainable rate, researchers at Purdue University are experimenting with specialized hardware aimed at offloading some of the AI demands on software.
The approach exploits features of quantum computing, especially proton transport.
“Software is taking on most of the challenges in AI. If you could incorporate intelligence into the circuit components in addition to what is happening in software, you could do things that simply cannot be done today,” stated Shriram Ramanathan, a professor of materials engineering at Purdue University, in an account from Purdue University published on sciencesprings.

The reliance on software with massive energy needs to make AI work is not sustainable, suggested Ramanathan. If hardware and software could share intelligence features, the silicon might be able to achieve more with a given input of energy.
The hardware the Purdue team is developing is made of quantum material, with properties the team is working to understand and apply to solving problems in electronics. The way software uses tree-like memory to organize information into various “branches,” is how the human brain categorizes information and makes decisions.
“Humans memorize things in a tree structure of categories. We memorize ‘apple’ under the category of ‘fruit’ and ‘elephant’ under the category of ‘animal,’ for example,” said Hai-Tian Zhang, a Lillian Gilbreth postdoctoral fellow in Purdue’s College of Engineering. “Mimicking these features in hardware is potentially interesting for brain-inspired computing.”
The team introduced a proton to a quantum material called neodymium nickel oxide. They discovered that applying an electric pulse to the material moves around the proton. Each new position of the proton creates a memory state; multiple electric pulses create a branch made up of memory states.
“We can build up many thousands of memory states in the material by taking advantage of quantum mechanical effects. The material stays the same. We are simply shuffling around protons,” Ramanathan said.
The team showed that the material is capable of learning the numbers 0 through 9, a baseline test of AI. The demonstration of these tasks at room temperature in a material is a step toward showing that hardware could offload tasks from software.
“This discovery opens up new frontiers for AI that have been largely ignored because implementing this kind of intelligence into electronic hardware didn’t exist,” Ramanathan said.
The results of this study are published in the journal Nature Communications.
Whether AI machine learning is making unreasonable power demands is also being investigated by John Naughton, professor of the public understanding of technology at the Open University, a public research university, the largest university in the UK for undergraduate education. He is the author of “From Gutenberg to Zuckerberg: What You Really Need to Know About the Internet.”

Researchers at Nvidia, the manufacturer of GPUs (graphic processing units) now used in most machine-learning systems, developed a natural language model that was 24 times bigger than its predecessor, and 34 percent better at its learning task, Naughton wrote in a recent account in The Guardian: Training the final model took 512 V100 GPUs running continuously for 9.2 days. One expert calculated that would be three times the yearly energy consumption of the average American.
“You don’t have to be Einstein to realize that machine learning can’t continue on its present path, especially given the industry’s frenetic assurances that tech giants are heading for an ‘AI everywhere’ future,” Naughton stated.
He, too, suggests that advances in hardware could help make the demands of AI more practical, noting that the new Apple iPhone 11 includes Apple’s A13 chip, which incorporates neural network software behind recent advances in natural language and image processing.
IBM Experimenting with The Neural Computer
Meanwhile at IBM, research is going on into the “neural computer,” a new type of computer designed to develop AI algorithms and assist in computational neuroscience. The Neural Computer is a deep “neuroevolution” system that combines the hardware implementation of an Atari 2600, image preprocessing, and AI algorithms in an optimized pipeline, according to a recent account in VentureBeat. (The Atari 2600, originally branded as the Atari Video Computer Systems, was introduced in 1977.)
The research team reports in an IBM technical research paper released earlier this year, that results so far have achieved a record training time of 1.2 million image frames per second.
Video games are a well-established platform for AI and machine learning research. In certain domains like reinforcement learning, the AI learns optimal behaviors by interacting with the environment in pursuit of rewards, such as good game scores. AI algorithms developed within games have been shown to be adaptable to practical uses, including protein folding prediction. If the results from IBM’s Neural Computer prove to be repeatable, the system could be used to accelerate the development of AI algorithms.
Over the course of five experiments, IBM researchers ran 59 Atari 2600 games on the Neural Computer. It required 6 billion game frames in total and failed at challenging exploration games like Montezuma’s Revenge and Pitfall. But it managed to outperform a popular baseline — a Deep Q-network, an architecture pioneered by DeepMind — in 30 out of 59 games after 6 minutes of training (200 million training frames). This compares to the Deep-Q network’s 10 days of training. With 6 billion training frames, it surpassed the Deep Q-network in 36 games while taking 2 orders of magnitude less training time (2 hours and 30 minutes).
Read the source articles in sciencesprings, The Guardian, VentureBeat and at IBM Research.