Artificial Intelligence
Unveiling the Importance of Transparent AI Algorithms in Criminal Justice Systems
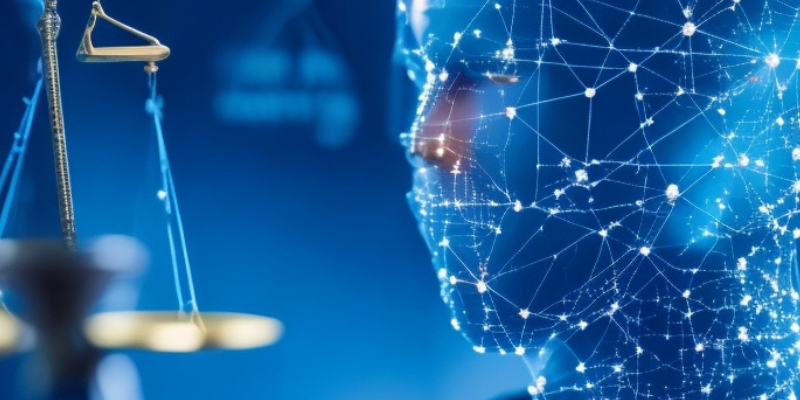
In an era where artificial intelligence (AI) algorithms have permeated every aspect of our lives, from social media feeds to the functioning of our legal systems, the need for transparency has never been more crucial. Particularly within the realm of criminal justice, where the stakes are high and the consequences profound, understanding and demanding transparency in AI algorithms is paramount. In this blog post, we will delve into the essential role of transparency in maintaining fairness and accountability in our legal systems and explore how the opacity of AI algorithms can perpetuate biases and erode public trust.
Introduction
In the era of artificial intelligence (AI), algorithms have become deeply integrated into our daily lives, influencing everything from our social media experiences to the functioning of the criminal justice system. As AI’s influence continues to expand, it is crucial that we prioritize understanding and demanding transparency in the operation of these algorithms, particularly within the realm of criminal justice.
AI Algorithms in Criminal Justice Systems
AI algorithms, often used interchangeably with artificial intelligence, are essentially sets of instructions designed to execute specific tasks such as pattern recognition or prediction-making.
There is an increasing utilization of AI algorithms in criminal justice systems worldwide. For instance, police departments employ predictive policing algorithms to identify crime hotspots and allocate resources accordingly. Courts adopt risk assessment tools that rely on machine learning algorithms to predict the likelihood of defendants reoffending.
Despite their growing prevalence, there remains a lack of transparency surrounding the inner workings of these AI algorithms. This lack of transparency poses serious implications for the fairness and accuracy of decisions made by criminal justice systems relying on them. Biases within algorithms can be magnified, resulting in unfair outcomes.
The Need for Transparency in AI Algorithms
Greater transparency is crucial to ensure the fair and accurate application of AI algorithms in criminal justice systems. Transparent practices would allow independent scrutiny of these algorithms, guaranteeing their fairness and accuracy. Moreover, transparency would enable public deliberation on the ethical implications of employing AI in this context.
Applications of AI in Criminal Justice Systems
AI algorithms are employed in various capacities within criminal justice systems. Predictive policing, risk assessment, and sentencing recommendations are some examples. Predictive policing algorithms utilize historical data to forecast future crime locations. Risk assessment algorithms evaluate an individual’s likelihood of reoffending. Sentencing recommendation algorithms employ past case data to suggest appropriate sentences for current cases.
While AI algorithms can be beneficial, there is a pressing need for transparency in their usage. These algorithms often rely on biased or inaccurate data, potentially perpetuating biases and disparities within the criminal justice system.
To address this issue, transparency should encompass the disclosure of training data, assumptions, and heuristics used in algorithm development. Additionally, ongoing monitoring and assessment of algorithm outputs are crucial to ensure fairness and accuracy.
Challenges with Traditional AI Algorithms
Traditional AI algorithms encounter several issues that can lead to inaccurate or biased results when implemented in criminal justice systems.
They are often designed and tested using non-representative data sets, which can result in good performance on artificial data but subpar performance on real-world data.
Traditional AI algorithms tend to be opaque, making it challenging to understand how they arrive at their results. This lack of transparency hampers the assessment of accuracy and the rectification of errors.
These algorithms often rely on a single input, neglecting the complexity of real-world decision-making situation. Such oversimplification can lead to biased or inaccurate outcomes.
Explainable AI: A Path to Transparency
As machine learning algorithms become more advanced, they are increasingly relied upon for high-stakes decisions in various contexts, including criminal justice. However, these algorithms are often opaque, potentially carrying the biases of their designers and operators. In the criminal justice domain, this lack of transparency can have severe consequences, exacerbating existing disparities and leading to wrongful convictions.
To address this issue, a movement toward explainable AI has emerged, aiming to make machine learning algorithms more transparent and understandable. Explainable AI algorithms are designed to provide human-readable explanations for their predictions, facilitating comprehension of how and why particular decisions are made.
Multiple approaches exist for designing explainable AI algorithms. Decision trees offer a concise breakdown of the algorithm’s decision-making process. Rule-based systems explicitly state the rules used to reach predictions.
While there is no perfect solution for achieving transparency in machine learning algorithms, explainable AI represents a promising step forward in ensuring responsible and equitable use of these powerful tools.
Benefits of Explainable Algorithms in Criminal Justice
Utilizing explainable algorithms in criminal justice systems offers numerous benefits. It enables a better understanding of the algorithm’s inner workings and the factors considered during prediction-making. This understanding helps prevent bias against specific individuals or groups. Additionally, explainable algorithms facilitate improved communication between different stakeholders within the criminal justice system, fostering alignment in decision-making. Ultimately, this can lead to fairer outcomes for all involved.
Challenges in Implementing Explainable AI
Implementing explainable AI in criminal justice systems presents several challenges.
AI algorithms are often complex and opaque, making it difficult to comprehend their decision-making process and ensure fairness.
Secondly, AI algorithms continually evolve, with regular updates and improvements by their developers. This dynamic nature makes it challenging to hold companies accountable for algorithmic decisions.
Imperfect data is a common issue in criminal justice. Incomplete or inaccurate data can result in erroneous decisions made by AI algorithms.
Conclusion
The critical and urgent need for transparency in AI algorithms used within criminal justice systems cannot be overstated. As we continue to advance this technology, we must bear in mind that real people’s lives are affected by the decisions made. Ensuring fairness and equity must be central to any decision-making process involving artificial intelligence.