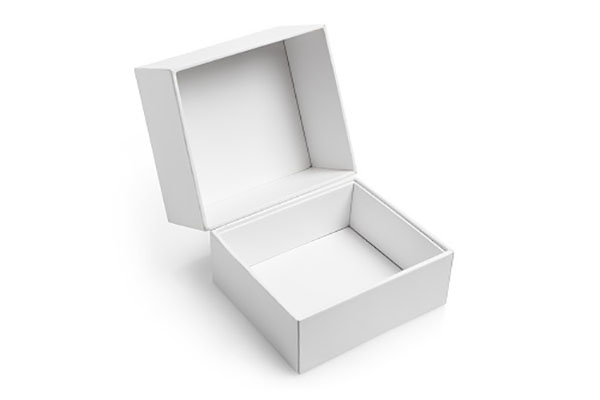
By Ryohei Fujimaki, Ph.D., Founder and CEO of dotData
AI is having a profound impact on customer experience, revenue, operations, risk management and other business functions across multiple industries. When fully operationalized, AI and Machine Learning (ML) enable organizations to make data-driven decisions with unprecedented levels of speed, transparency, and accountability. This dramatically accelerates digital transformation initiatives delivering greater performance and a competitive edge to organizations. ML projects in data science labs tend to adopt black-box approaches that generate minimal actionable insights and result in a lack of accountability in the data-driven decision-making process. Today with the advent of AutoML 2.0 platforms, a white-box model approach is becoming increasingly important and possible.

White vs. Black: The Box Model Problem
White-box models (WBMs) provide clear explanations of how they behave, how they produce predictions, and what variables influenced the model. WBMs are preferred in many enterprise use cases because of their transparent ‘inner-working’ modeling process and easily interpretable behavior. For example, linear models and decision/regression tree models are fairly transparent, one can easily explain how these models generate predictions. WBMs render not only prediction results but also influencing variables, delivering greater impact to a wider range of participants in enterprise AI projects.
Data scientists are often math and statistics specialists and create complex features using highly-nonlinear transformations. These types of features may be highly correlated with the prediction target but are not easily explainable from the perspective of customer behaviors. Deep learning (neural networks) computationally generates features, but such “black-box” features are understandable neither quantitatively nor qualitatively. These statistical or mathematical feature-based models are at the heart of black-box models. Deep learning (neural network), boosting, and random forest models are highly non-linear by nature and are harder to explain, also making them “black-box.”
WBMs and Impact on User Persona
There are three key personas to consider when applying ML to solve business problems: model developers, model consumers and the business unit or organization sponsoring ML initiative. Each persona has a different priority and implications based on the specific modeling approach. Model developers care about explainability, model consumers care about actionable insights and for companies and organizations, the most important attribute is accountability:
- Model developers and explainability: Model developers need acceptance from business users and must be able to explain model behavior to business functions or regulators. Hence explainability is critical for model acceptance. Model developers have to explain how their models work, how stable their models are, and which key variables drive decision making. WBMs produce prediction results alongside influencing variables, making prediction fully explainable. This is especially critical in situations where a model is used to support a high-profile, high-impact business decision or to replace an existing model, and model developers must defend their models and justify model-based decisions to other business stakeholders.
- Model consumers and actionable insights: Model consumers are using ML models on a daily basis and need to understand how and why a model made a particular prediction, to better plan how to respond to each prediction. Understanding how a score has been derived, and what features contributed, allows consumers to optimize their operations. WBMs explain influencing variables and their impact on prediction results. This helps model consumers, who are typically business users, take actions towards the high-importance influencing variables, directly changing business outcomes. For example, suppose a black-box model indicates that “Customer A is likely to churn within 30 days with a probability of 73.5%. Without a stated reason for the likely churn, a salesperson will have insufficient information to determine if the prediction is reasonable, and hence, how much confidence to give to the prediction in question. WBMs give a different answer, such as, “Customer A is likely to churn next month because Customer A contacted the customer service center four times in the past 30 days, and the service usage from Customer A decreased by 20% during the past three months.” This detailed explanation makes it easier for model consumers to determine the validity of the prediction. This type of model also suggests that ‘number of times a customer contacts customer service center’ and ‘service usage for the three months’ could be strong indicators of customer churn probability and thus should be closely monitored to prevent similar customer churns.
- Organization and accountability: Companies always need accountability to help mitigate and manage risk. Controlling model behavior is critical to ensure that the appropriate information is used and that models are within compliance boundaries. WBMs allow businesses to maintain a higher degree of accountability with how ML is being used in data-driven decision-making. As more organizations adopt data science to optimize business processes, there are increasing social concerns about decisions made based on personal or potentially discriminatory information. For example, in loan applications, race and gender should not be used to determine consumer eligibility. Black-box models exacerbate this issue, where less is known about the influencing variables actually driving the final decision. WBMs help organizations stay accountable for their data-driven decisions and comply with the law and legal audits.
Transparency Levels
It’s very important for analytics and business teams to be aware of the varying levels of transparency and their relevance depending on the nature of the business.
In principle, Black Box transparency means analyzing input-output relationships. With black-box models, it’s impossible to gain insight into what’s happening inside the model but you can observe the output for any given input. Based on this information and repeating trials, observers can see how input impacts output. This is the lowest level of transparency. Model consumers don’t know how the model uses different inputs and determines results. This level provides an insufficient amount of transparency for any business.
White Box transparency means that the exact logic and behavior needed to arrive at a final outcome is easily determined and understandable. Linear and decision tree models are intrinsically easy to understand and White Box. Recently, there are studies on techniques to approximate Black-Box models by a simpler model and try to explain Black-Box models. However, practitioners should remember that a highly-nonlinear model in a very high dimensional space is essentially hard to even approximate, and there is non-ignorable risk to rely on such an approximation technique if transparency really matters.
Interpretability, however, implies that there is a much deeper and broader level of understanding. In other words, does the model make sense for business? Feature interpretability comes to be extremely important because it is impossible to give clear business interpretation to highly nonlinear feature transformation even if a ML model itself is white-box.
AutoML and White-Box Modeling
AutoML is gathering momentum. The most advanced platforms (a.k.a. AutoML 2.0) even automate feature engineering, the most time consuming and iterative part of ML. AutoML significantly accelerates AI/ML development and implementation for enterprise and empowers a broader base of professionals like BI experts or data engineers in the development of AI/ML projects.
Since the major part of FE and ML modeling process is automated, model and feature transparency is even more critical to implement AutoML in organization. Automated FE automatically discovers hypotheses of useful data patterns via statistical algorithms. Since there is little intervention of domain experts, domain/business interpretations have to be given, retrospectively. In other words, features generated by AutoML 2.0 must have understandable representation for human experts. Such transparent features lead to interpretable model behavior.
Summary
Today’s data science applications require white-box models. As more organizations adopt data science into their business processes, there are increasing concerns and risks about automated decisions made by ML/AI models. Interpretable features help organizations stay accountable for their data-driven decisions and meet regulatory compliance requirements. With WBM data science is actionable, explainable and accountable. AutoML 2.0 platforms along with WBMs empower enterprise model developers, model consumers and business teams to execute complex data science projects with full confidence and certainty.
Ryohei Fujimaki, Ph.D. is the Founder & CEO of dotData, a leader in full-cycle data science automation and operationalization for the enterprise. Prior to founding dotData, he was a research fellow for NEC Corp. He was instrumental in the successful delivery of several high-profile analytical solutions now widely used in the industry. Ryohei received his Ph.D. degree from the University of Tokyo in the field of machine learning and artificial intelligence.