Artificial Intelligence
AI Vs Machine Learning – What’s the Difference?
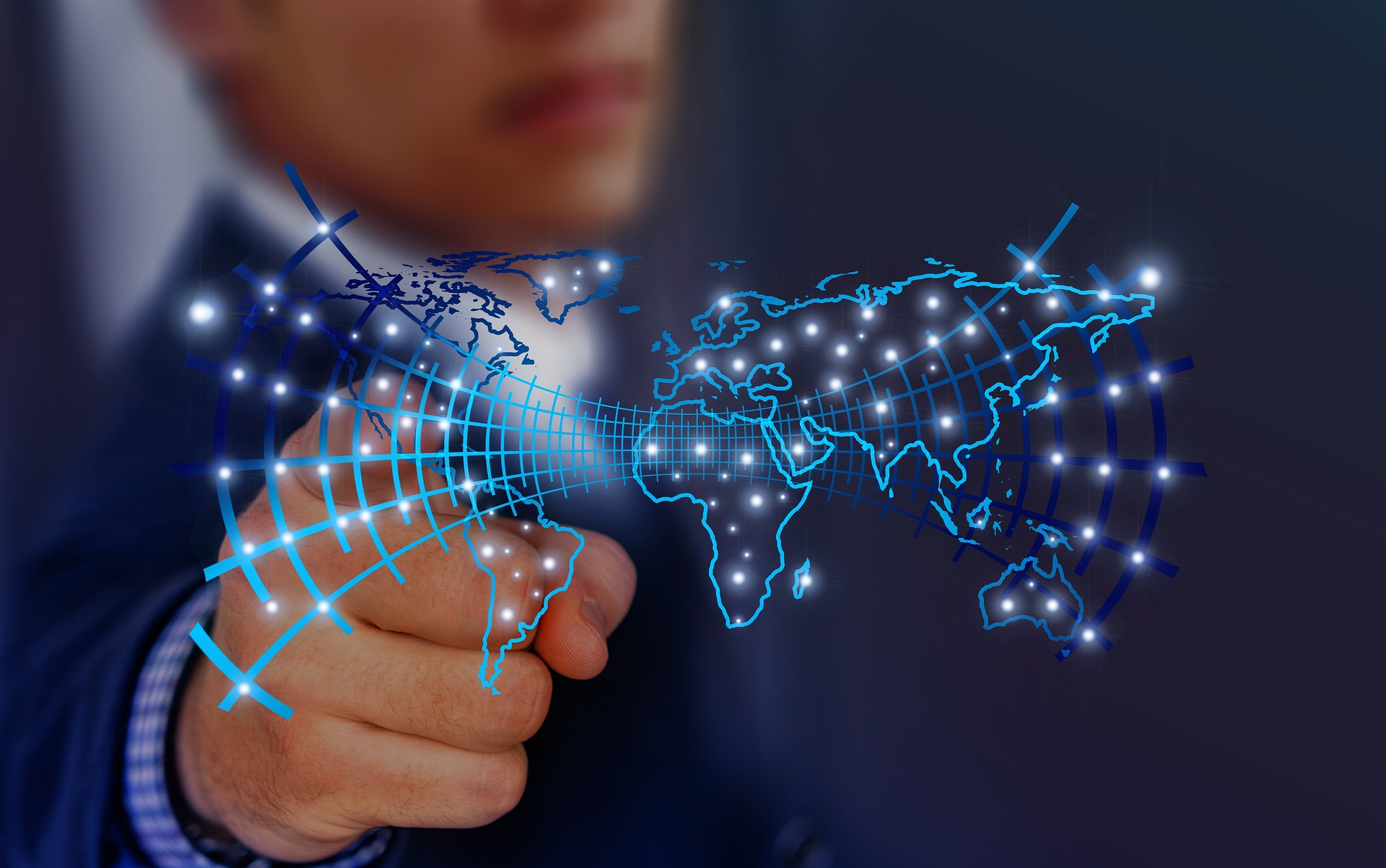
Many businesses are turning to artificial intelligence (AI) and machine learning technologies to increase efficiency, gain valuable insights, and automate processes. But there are several key distinctions between them.
Machine learning (ML) models use data sets to recognize patterns and optimize performance for certain tasks, like recommendations or sentiment analysis. If biased information or data reflecting existing inequities are fed into these algorithms, they could perpetuate discrimination.
Cost
AI is an exciting technology with great promise to transform businesses by automating internal processes and improving customer experiences. However, adopting AI comes at a price, which may be difficult to estimate due to numerous variables including hardware costs, labor expenses, and data processing fees – although recent trends suggest its costs have been declining considerably.
Costs associated with AI implementation depend on the project type and level of accuracy required; higher-level AI projects tend to be more expensive. For instance, customer support chatbots that handle complex questions may need to be trained by human specialists – increasing their implementation costs significantly.
Another factor influencing AI costs is data availability and quality. More accurate results are achieved with more data available for training; however, collecting that data can be costly when external sources don’t provide access. Furthermore, unstructured information may require costly processing solutions.
Hardware used to run AI algorithms also has an effect on costs; generally speaking, more powerful machines may be required in order to support AI applications – something which may restrict availability for smaller businesses.
Accuracy
People frequently conflate artificial intelligence (AI) and machine learning (ML). While AI aims to mimic intelligent behaviors, ML algorithms focus more on accuracy. They detect patterns in data to pinpoint trends for automation purposes and improved customer service.
However, AI and ML differ considerably in their scope and applications; AI provides much broader uses than ML such as image recognition or natural language understanding. Furthermore, it’s able to make decisions without being programmed directly – making it an invaluable asset for businesses.
Machine learning (ML) is an emerging subfield of AI that strives to mimic human intelligence in machines. Machines able to learn from past experiences, predict future patterns, and constantly improve over time are often employed as part of company savings efforts by streamlining manual processes and increasing productivity.
While machine learning (ML) can simplify many tasks, it is not infallible. When dealing with large amounts of unstructured data, finding accurate patterns may prove challenging. Therefore, to ensure successful ML training results it is vital that organizations implement systems for managing and protecting this sensitive data such as health records or credit card numbers.
Time
Machine Learning (ML) is an innovative field encompassing various technologies. ML applications range from recognizing objects in images to making recommendations that meet customer needs; speech recognition, natural language understanding, and sentiment analysis also fall under its purview. Businesses can utilize these applications to increase business operations efficiency while improving return on investment.
As opposed to traditional programming, which involves giving computers precise instructions, machine learning (ML) models are trained by themselves by following patterns in data. This makes it simpler for machines to become adept at performing certain tasks without human assistance; however, this process may take considerable time when dealing with large volumes of information and can sometimes result in unintended results.
Artificial Intelligence can help companies streamline various aspects of their business, including customer support. AI can reduce operational costs by decreasing employee labor requirements for certain tasks and can detect and respond more rapidly to customer problems; ultimately leading to higher profits and improved customer satisfaction.
Even with all of the buzz surrounding AI, many companies still struggle with its implementation. One major roadblock for implementation is lack of staff skills – which 56% of companies report having to contend with. There are ways around this obstacle, including providing training courses or encouraging employees to keep abreast of technological innovations.
Limitations
Many people mistake artificial intelligence (AI) and machine learning (ML), but these two technologies have different scopes and applications that have significant ramifications for business operations. Understanding the differences can have lasting repercussions for any organization.
Modern artificial intelligence algorithms differ significantly from their predecessors in that they’re designed to become increasingly accurate over time, offering greater functionality than rule-based systems that have limited capabilities. AI is used in various fields including self-driving cars and power grid optimization; however, there may be certain restrictions with AI that must be considered.
Machine learning models may have severe social and ethical repercussions when used to make decisions that affect people’s lives, particularly if their training data is biased and can perpetuate or even amplify existing biases and discrimination. Furthermore, machine learning models may have difficulty accurately interpreting human behaviors like tone or emotion interpretation and this renders them unsuitable for certain applications such as credit card fraud detection.
Machine learning algorithms may also be susceptible to errors due to the large number of trials involved. For instance, learning to recognize faces in photos or understand complex sentences takes much training data, not to mention computing resources that may prove too expensive for smaller businesses.